What is ProjectPredict?¶
ProjectPredict is a library to help project managers gain insight into the status of their project using Bayesian networks. It is inspired by the paper “Project scheduling: Improved approach to incorporate uncertainty using Bayesian networks” (Khodakarami, Fenton, & Neil, Project Management Journal, 2007). The project features
- Inferring the latest start date, earliest finish date, and total float for each task in a project
- Recommending which task or tasks should be started next using custom constraints and objective functions
- Task duration specified either through three-point (PERT) estimation or inferring the duration of a task from a machine learning model
- Visualization of a project timeline using Matplotlib
The Bayesian network¶
A project is specified as a directed acyclic graph of tasks. For example, suppose you have three tasks, A, B, C, D, E, and F. Task C can only be begun when tasks A and B are completed, task D can only be completed when task B is completed, and tasks D and E can only be begun when task B is completed. The resulting graph would look like this:
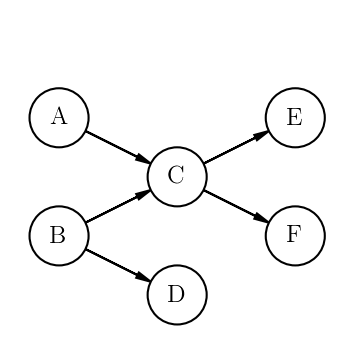
Each task is then decomposed into a smaller Bayesian network.
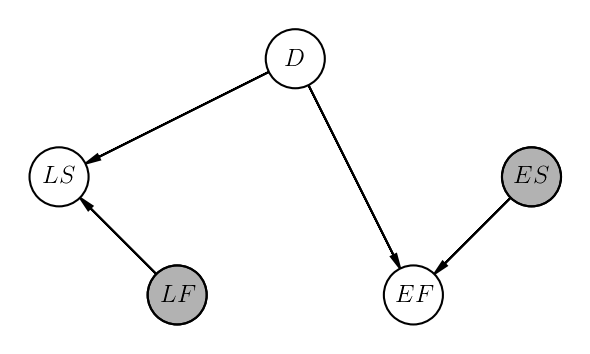
Where \(D\) is the duration, \(ES\) is the earliest start date, \(LS\) is the latest start date, \(EF\) is the earliest finish date, and \(LF\) is the deadline or latest finish date. The earliest finish date can be inferred from the graph by traversing the graph in topological order from the starting tasks (A and B in our example), from the equations
\(ES_i = \max \{ES_j + D_j \; \forall \; \text{predecessor tasks}\; j\}\)
\(EF_i = ES_i + D_i\)
The latest start date for each task can be inferred by traversing teh graph in reverse topological order from the final tasks (D and E in our example), from teh equations
\(LF_i = \max \{LF_j - D_j \; \forall \; \text{successor tasks}\; j\}\)
\(LS_i = LF_i - D_i\)
For our sample project, tasks A and B must be given an earliest start date, and tasks C and D must be given a latest finish date. Both of these can take the form of either a probability distribution or a hard date. All tasks must be given a duration, either using three-point estimation or predicted from a learning model.
Once these values have been inferred for each task, the total float can be defined as \(TF_i = LF_i - EF_i\). This is a measure of the amount of time a task’s duration can be increased without affecting the completion time of the project as a whole. The smaller the total float of a task, the more critical the task is to the overall project.